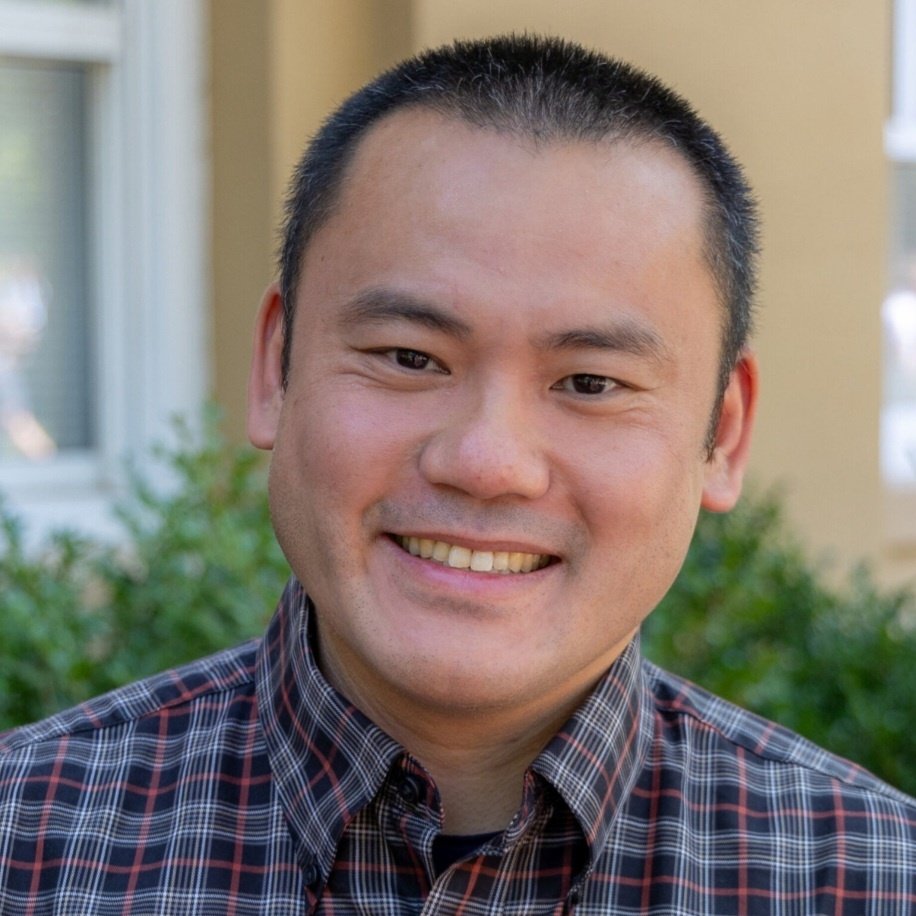
My research group conducts research in the following areas of statistics and machine learning:
- methods and scalable algorithms for high-dimensional data
- deep learning and deep generative models
- Bayesian methodology and computation
- survival analysis and causal inference
- reinforcement learning and explainable artificial intelligence
- precision medicine, clinical trials, drug discovery, GWAS, and other biomedical applications.
Prospective Students
To work with me, you must be currently enrolled as a student at USC. PhD students who are interested in my supervision must also first pass the USC Statistics PhD Qualifying Exam (offered every August). Information for prospective studentsIf you are interested in applying to the Statistics graduate program at USC but are not yet enrolled there as a student, please visit the department webpage for information about applying. Note that graduate students are admitted by the department’s Graduate Admissions Committee — not by myself or individual faculty. I am also unable to provide specific feedback on anyone’s application or recommend applicants whom I do not know for admission.
Recent News
- June 2024: “Bayesian modal regression based on mixture distributions” (with Qingyang Liu and Xianzheng Huang) has been accepted by Computational Statistics & Data Analysis. Paper
- June 2024: New preprint “Quantifying patient and neighborhood risks for stillbirth and preterm birth in Philadelphia with a Bayesian spatial model” (with Cecilia Balocchi, Jessica Liu, Silvia P. Canelón, Edward I. George, Yong Chen, and Mary R. Boland). arXiv
- June 2024: New preprint “Neural-g: A deep learning framework for mixing density estimation” (with Shijie Wang, Saptarshi Chakraborty, and Qian Qin). arXiv
- May 2024: My student Shijie Wang has defended his PhD dissertation “New Deep Learning Approaches to Classical Statistical Problems” and will join Gauss Labs as an Applied Scientist. Congratulations, Shijie!
- March 2024: “Fast bootstrapping nonparametric maximum likelihood for latent mixture models” (with Shijie Wang and Minsuk Shin) has been published in IEEE Signal Processing Letters. Paper
- February 2024: “Generative quantile regression with variability penalty” (with Shijie Wang and Minsuk Shin) has been accepted by Journal of Computational and Graphical Statistics. Paper
- January 2024: New preprint “A unified three-state model framework for analysis of treatment crossover in survival trials” (with Zile Zhao, Ye Li, and Xiaodong Luo). arXiv